Buyer’s Guide to AI Learning Products
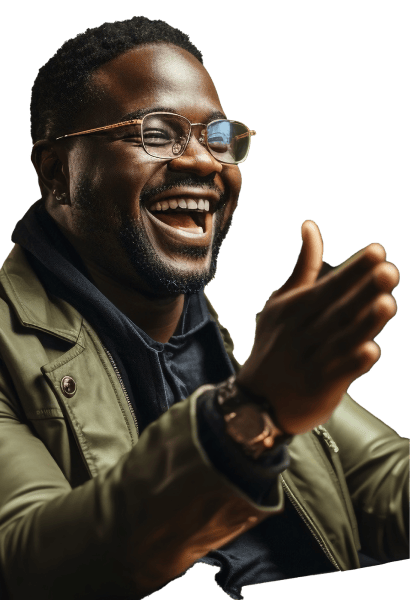
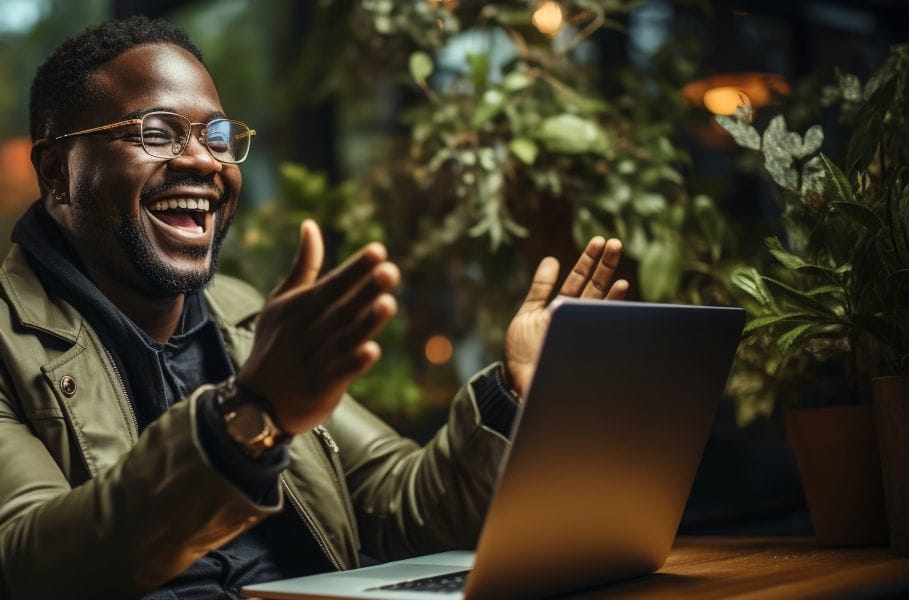
01
Contributors
Michael Chong
Senior Data Scientist (Docebo)
Rebecca Chu
Machine Learning Analyst (Docebo)
Vince De Freitas
Product Marketing Manager, Content & AI (Docebo)
Maija Mickols
AI Product Manager (Docebo)
Giuseppe Tomasello
Vice President of AI (Docebo)
Renee Tremblay
Senior Content Marketing Manager (Docebo)
02
How to use this guide
Artificial Intelligence (AI) has become increasingly essential for L&D teams seeking to enhance training programs, engage employees, drive organizational success, and stay competitive. But are all AI learning products created equal? Probably not.
So how do you differentiate between what’s hype and what’s actually helpful?
That’s where this guide comes in. The Buyer’s Guide for AI Learning Products is intended to assist L&D professionals as they navigate the learning AI landscape, offering valuable insights, and helping you make informed decisions when considering the purchase of AI solutions for your learning initiatives.
Whether you’re exploring AI’s potential to personalize learning experiences, automate administrative tasks, or optimize content delivery, this guide will provide you with a roadmap to identify and select AI tools that align with your organization’s unique learning and development needs.
03
The evolving landscape
AI is quickly becoming an integral part of business. According to recent research, 35% of global companies report using AI in their business.
But it isn’t just availability of AI (or the novelty) that’s driving this rapid adoption. AI is quickly becoming a necessity due, in part, to two macro trends that are significantly impacting the workforce.
- The relationship between the population growth rate and unemployment
- Drastically changing demands and expectations of L&D teams
Let’s start with the annual population growth rate—or, more specifically, the lack thereof.
Global birth rates have dramatically declined. According to The Economist, the largest 15 countries (by GDP) all have a fertility rate below the replacement rate, which means people are aging out of the workforce faster than we can replace them.
It used to be that there were more unemployed people than job openings. But over the past few years, that paradigm has flipped, and now there are more open jobs than people to fill them. All this adds up to a labor shortage, which leads to increased competition for skilled workers.
Demographics aren’t the only thing dramatically evolving. The learning landscape is also changing.
It’s become much bigger.
Ten years ago, the majority of use cases for training were internal (e.g. onboarding, talent development, compliance, etc.). That meant fewer programs being delivered to a smaller number of learners. Today, more organizations are extending learning outside of the enterprise. Docebo’s internal customer data reflects this evolution.
This isn’t unique to our organization. It’s indicative of a larger trend. Studies, including a recent one from Brandon Hall Group, show that more than 50% of organizations deliver learning to external, non-employee groups. These include customers, channel partners, distributors, value-added resellers, and franchisees.
Once training extends outside of the organization, the number of learners (along with the programs and content needed to support them) grows exponentially.
04
The promise of Artificial Intelligence (AI)
Fewer people. More work. That’s our current reality. And it’s a huge part of what’s making AI such an appealing solution.
As the diagram below shows, AI is outpacing human performance in many areas, including image recognition, reading comprehension, and language understanding. This presents organizations with a solution to offset talent shortages, while increasing efficiency by offloading a lot of the repetitive, uncreative tasks.
Forrester predicts that enterprise AI initiatives will boost productivity and creative problem-solving by 50%. Not only can incorporating AI into the business help mitigate the labor shortage, but it can also deliver competitive advantage. According to 2023 data, AI saves an employee 2.5 hours per day on average.
While AI solutions hold a great deal of potential and promise, it’s important to remember that an AI product for one part of the business might not be helpful for another. For example, an AI-powered contract review solution might increase productivity and efficiency for your Legal team, but it isn’t going to do much to help the Learning and Development team. In other words, not just any AI solution will do. You’ll need purpose-driven AI products across your organization, and L&D teams will need learning AI solutions.
Did you know
In 2008, developers created a chess-playing AI called Stockfish and taught it the rules and strategies of the game. Running at full power, it’s nearly impossible for a human to win against Stockfish. In December 2017, a competing AI called AlphaZero was created. This AI was never taught the strategy, but simply trained itself by observing a massive number of chess games and playing practice games against itself for a few days. With equal computational power behind each model, AlphaZero beats Stockfish consistently.
05
What is AI for Learning?
When we talk about “AI for Learning,” or “Learning AI,” we’re talking about AI products, models, and agents made specifically to support instructional designers, L&D teams, and other learning professionals. In the larger context of AI, today’s learning AI products generally fall under the Natural Language Processing (NLP), speech, and vision branches—all of which make use of machine learning.
When evaluating learning AI solutions, a more helpful and perhaps practical way to look at learning AI is in terms of what it does—the tasks it can carry out. More specifically, what tasks it can do that are relevant to your L&D organization. That’s where the true opportunity lies.
Maybe you’re having a hard time hiring Instructional Designers, so your team is falling behind in content creation. Perhaps the demands on your team outpace your production capacity. You could have a skills gap on your team, so you’re unable to analyze data. Or maybe you’re scaling your programs or business and require translation capabilities. Ultimately, a learning AI should help you solve a particular problem. Therefore, your decision should be problem driven, not product driven.
Here’s a tip
A good approach is to treat a potential AI purchase like you would a job candidate. (You’re not buying a product; you’re hiring help!) You wouldn’t hire someone just because they’re well-dressed and available. So you shouldn’t buy an AI product because it’s smart and shiny. Like humans, AI tools have limitations. With candidates, you uncover these limitations during the interview process. The product evaluation is your opportunity to suss out potential limitations in the AI and make sure your processes include checks and balances that mitigate risks associated with those limitations.
06
Basics concepts of Learning AI
In order to make an informed decision when buying learning AI products, there are a few basic concepts you should be familiar with.
Levels of learning design automation
As you introduce AI into your organization, it’s important to think about the intersection between AI and human-driven learning design. As the table illustrates, as you seek deeper levels of automation, there’s a sliding scale between the human and the machine as it relates to matters of control, personalization, and outputs.
On the left, you’ve got a heavy hand of control against the system that you’re using. Actions and decisions will be driven by the content or instructional designer—and the enhancements and automation from AI will be limited at best. As you move across the scale to the right however, a greater degree of action taking and decision making is released to the AI system. In return, you get a much greater depth of personalization, automation, time savings, and scalability of your learning program.
The benefits of deeper automation go beyond just the items listed above. For most functions within a business, there always exists a certain amount of repetitive, manual bits of work or tasks that actually interfere with productivity. This could be summarizing or reorganizing data. It could be writing quiz questions. It could be that one time you had to spend hours and hours relabelling metadata because it messed that one table up.
Toil is an invisible, insidious part of your work life that artificial intelligence can manage with ease. If you spend 20% of your day wrestling with tedious toil, AI can help you regain that time and help you get ahead or, sometimes, just catch up.
None of that happens at Level 1 on the table above, though. To regain your time and delegate to AI, you must first understand the problem that you’re trying to solve, and then find a solution (like machine learning-powered content curation, data analysis, AI-based skills mapping, or Generative AI content creation tools) that addresses that problem.
Speaking of Generative AI, now would be a good time to unpack what it is and how it works at a high level.
07
Generative AI (GenAI) explained
Prior to Generative AI, artificial intelligence typically specialized in recognizing and predicting things. It could have been turning the squiggles from a scanned document into editable text, or taking an audio file and transcribing words from the sound waves. On smartphone keyboards, basic types of artificial intelligence could attempt to predict or guess your next word. With Generative AI however, artificial intelligence creates things based on patterns it recognizes in source data.
Did you know
Generative AI is able to write a sonnet in the style of William Shakespeare. Shakespeare was incredibly consistent, and it helps that he put his name on most of the sonnets he wrote. GenAI can also create art in the style of Vincent Van Gogh. This opens up a wide array of exciting possibilities, but also ethical considerations around the copyright of the artists whose work ends up used in these AI models without explicit consent.
At its core, GenAI leverages deep learning AI models to study vast libraries of data. This could be every image and piece of written text in the public domain, all public-facing social media, news articles, and more. As AI companies feed more data into their models, the accuracy and reliability of these models increases. As it intakes data, it begins to recognize patterns. These patterns can include things that humans take for granted, or feel like we just know.
It could include the way light is supposed to bounce off a person’s eye in a photograph. It could be the predictable structure that nouns and verbs fall into in most sentences. It may even be the proper way to pronounce “emphasis.” These are the types of patterns that we don’t think of consciously, but will immediately recognize if something isn’t the way we expect it. After training on millions, if not billions of documents, images and videos—it turns out AI can recognize those patterns too, especially if everything you’ve given it is consistent in how it does those things.
Generative AI interprets the consistency of these patterns as an expectation of what humans do, and expect to see. In a lot of ways, it just wants to give us what we want. We simply have to be intentional about the data used to train AI, when it processes it all, and at the end of all of that, we often get something really, really close to what we expected or could have done ourselves.
Here’s a tip
Not sure if the image you’re looking at is real or AI-generated? If there’s a person in it, check out their hands. Reference data for hands is typically limited in non-specialized image-generation models and are often one of the first things to look off, or funky. If an image features text or logos, take a closer look to see if they appear unnatural. AI-generated text can appear pixelated or stretched, and logos may be altered.
08
Potential challenges with AI solutions
AI can feel a bit like magic. You can get results without knowing or understanding how the AI arrived at them. That’s not ok. This isn’t Oz. We need to pay attention to what’s happening behind the curtain—or, in the case of AI, what’s happening inside the black box.
Did you know
According to a recent study, 86% of users surveyed have experienced AI hallucinations when they use chatbots like ChatGPT and Bard. Despite this, 72% still trust the AI.
09
Frameworks for successful AI
There’s no quick fix for the black box problem, hallucinations, bias, and other issues inherent in AI, but thankfully there are a number of smart people and organizations working on this problem. While developing AI-powered solutions, the Docebo team dug deep into our own research and found these principles, techniques, and frameworks to be helpful when thinking about effective, learner-centric, and reliable solutions.
Did you know
Andragogy or Pedagogy? Andragogy refers to the best practices and research-backed methods for teaching adult learners, and pedagogy typically refers to the best practices and research-backed methods for teaching children. However, pedagogy is often used in a broad context to describe methods for both adults and children alike (eg. colleges and universities often use pedagogy over andragogy). When we use the term pedagogy, it’s in the broader sense and acknowledges that our learners are adults.
From human processes to AI frameworks
Whether you work with graphics, authoring, or instructional design, there’s a creative process behind your craft. These processes are repeatable, situational, and foundational to the types of artifacts that you create. They can also be replicated and trained to an AI to help improve your workflow, giving you time back to focus on bigger picture strategic decision making. Less toil, more productivity.
The diagram below examines one way to translate some of the strategic decisions that an instructional designer makes while writing multiple-choice questions (MCQs) into a framework that AI can follow to deliver similar or improved results in a fraction of the time.
While writing MCQs, there are a lot of different factors that instructional designers take into account that affect the quality of assessment.
Using Docebo’s methodology, we capture all these factors and use them as input variables to generate an output that embodies all these otherwise human decisions. Note that the sequence of decisions is also in line with the order in the human authoring process and the dependencies of each decision. For example, when writing a reading comprehension activity, human authors would write a reference text first and then write the stem (question), and finally the options. This is exactly the order of how the prompt chains will be executed in the generation process.
Today’s AI for learning still needs to be coached by the crafter. As such, the most effective learning products will have human methodologies (like the one illustrated above) behind them to allow them to think and make decisions like an instructional designer.
10
Next steps
Now that you know more about AI for learning, you’re ready to start your search. But, before you begin, we suggest you answer these six questions to help guide you and your team as you evaluate potential vendors and shop for AI learning products.
6 big questions to answer before engaging with vendors
- What’s driving your buying decision? Is this product acquisition based on solving an existing problem, or do you have leftover budget and the demo was shiny?
- How will this impact the user experience? Does this product build on existing user behavior, or does it expect users to learn new skills? (And what does this mean for onboarding and time-to-value?)
- Who is in control of data and process? Who decides what data is relevant to the AI model? Where will your data be stored and processed?
- How will you avoid the black box problem? Can you inspect, explain, or override the AI model’s decisions, if needed?
- Is learning central to the AI model? Will pattern recognition drive the creation of learning materials or are pedagogically effective strategies built in to guide the AI processes?
- How will you manage this product? Artificial Intelligence (AI) products rely on the quality of its inputs. How will you ensure that the content it consumes is real, relevant and reliable?
11
About Docebo AI
We believe that artificial intelligence provides one of the most powerful opportunities for innovation in learning and development. As leaders in this space, we don’t follow trends, we create them. Docebo’s learning platform is powered by artificial intelligence, with several AI features included throughout the system to enhance the way businesses and enterprises deploy, manage, and scale their learning programs.
Here are a few guiding principles for how we think about AI in our products and services, and what you can expect from Docebo AI.
A pedagogy-first approach: When it comes to learning and development, an AI framework that doesn’t include pedagogy and established best practices in teaching and learning just won’t cut it. Our AI product team includes pedagogical experts who use a pedagogy-first approach to designing our AI solutions to maximize learner outcomes while minimizing hallucinations and inaccurate results.
Continuous assessment: We’re building a flywheel of robust continuous learning and assessment to help inform our AI models. When AI models rely solely on pattern recognition and replication, the results are not suitable for learning content. By understanding and leveraging learner performance through integrated assessment, the learning flow is never interrupted and a more comprehensive perspective is maintained. Docebo AI is connected to the bigger picture and provides the fundamental backbone for hyper-personalized learning.
Reaching towards individualized learning: Hyper personalization and learning in the flow of work are inevitable outcomes of AI in learning. At Docebo, we’re focused on delivering this to our customers through smart implementation of safe and effective AI.
Inspectable, Explainable, Overridable: An effective AI for learning solution never removes humans from the process and its outcomes. At Docebo, we design with inspectability, explainability and overridable at the heart of our products and services, empowering the user to be in control and have confidence in their work.
Freeing you up to focus on what matters: Docebo’s intent is to design AI learning solutions that offload much of the repetitive and time-consuming work, so learning designers can focus on what really matters, like data interpretation, content governance, strategic decision making, and holistic learning design.
Giving you control: Organizations should be in control of how AI functions within their business. Docebo’s solutions provide businesses with control over their own data so they can decide how (and if) it is used. We also provide data anonymization on our own LLM to ensure data privacy and control.
Want to explore how a GenAI learning platform can transform your business?